Along with information technology advances in recent years, the time has come where the technology to adequately collect and manage information, and effectively utilize analytical findings is necessary in every domain.
The importance of data science is widely recognized in academia as a fourth paradigm for driving research through the collection and analysis of massive amounts of data.
In the industrial domain, effective data accumulation and utilization to create value has taken on an ever-larger role in strengthening competitiveness.
Rapidly advancing artificial intelligence technologies are reaching a level that outstrips human recognition and decision-making capabilities in some areas.
The changes in society with such technologies in the background are also driving reforms in the type of people universities should be producing.
With this in mind, the University of Tsukuba has established the Data Science Literacy Program (“DSL” hereafter).
It consists of two compulsory subjects for all first-year students, namely Information Literacy, which aims to help students acquire fundamental information literacy and the skills to use computers, and Data Science, which helps them develop objective decision-making skills based on data.
Our DSL program is certified as an “Advanced Program for Mathematics, Data Science and AI Smart Higher Education” established by the Ministry of Education, Culture, Sports, Science and Technology (MEXT) with the goal of improving fundamental mathematics, data science, and AI-related skills and offering greater opportunities in such areas.
It has been selected as an “Approved Program for Mathematics, Data Science and AI Smart Higher Education,” which indicates programs with unique, leading initiatives.
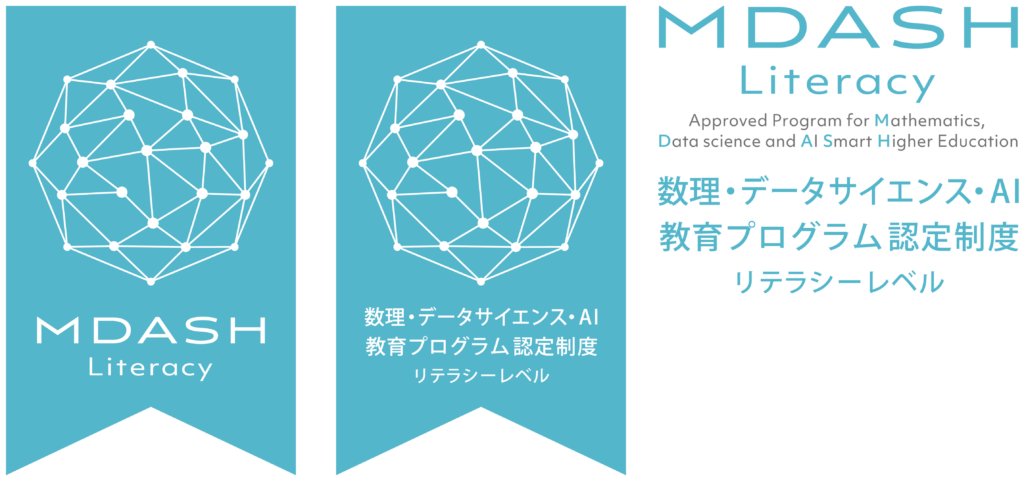
(Certification expiration: March 31, 2026)
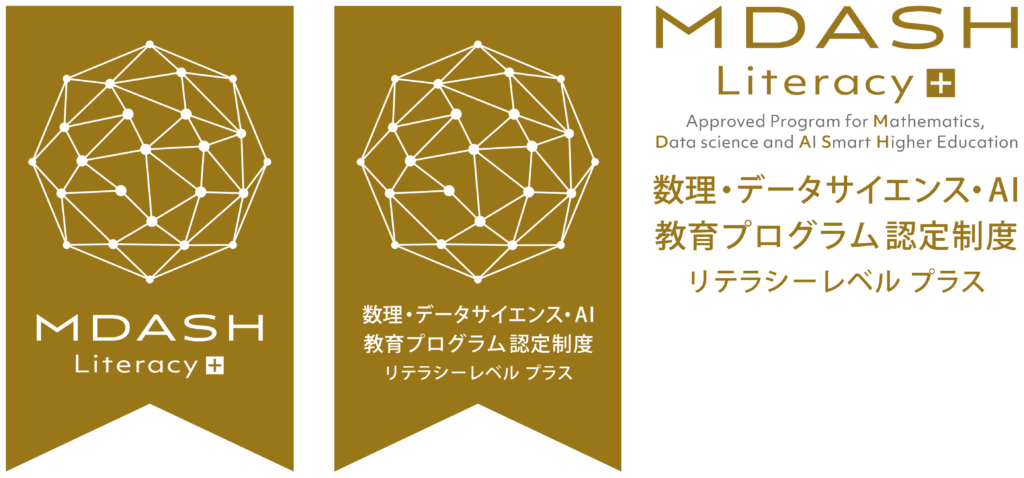
(Certification expiration: March 31, 2026)
References
MEXT “Approved Program for Mathematics, Data Science and AI Smart Higher Education” (Japanese only)
DSL Program Features
Feature (1) 100% completion rate of DSL program upon graduation since 2019
The University of Tsukuba is home to students from a wide variety of academic disciplines unlike any other school, not only just in literature and the sciences, but in physical education and the arts.
Since 2019, Information Literacy (lecture) and Data Science have been compulsory subjects for first-year students in all disciplines.
With all first-year students in mind, course materials compatible with all bachelor programs, not only on science and engineering tracks, but also in literature, physical education, and the arts, and that meet the needs of society are created and used across the school.
Feature (2) A curriculum that focuses on practical execution
By combining lectures and exercises, the DSL program provides classes where students can learn in a more practical fashion.
This is a comprehensive educational program covering the entire life cycle of data science, from statistical analysis to data collection, management, and analysis, along with a curriculum that focuses on practical execution centered on practice utilizing real world data.
Please take a look at the curriculum for further details.
Feature (3) Course material design based on evidence (assessment of educational effectiveness, etc.)
Assessment of educational effectiveness does not end with class evaluations by students, rather, motives for learning data science and an objective evaluation of understanding are taken into account. Information on class content, desire for learning, and retention rate gleaned from questionnaires on lecturers including those with work experience at private enterprises is collected and used in the common subject Information Promotion Office to improve course materials.
Our curriculum is arranged so that students that are unfamiliar with data science, those that wish to engage in more advanced studies, and students in a variety of disciplines can find strong motivation to take on studies in data science.
Feature (4) Introduction videos in various disciplines for sparking interest and motivating a diverse range of students
For first year students encountering data science for the very first time, video lectures by faculty in various fields introducing examples of data science in a variety of academic disciplines are incorporated into the curriculum.
For first year students encountering general topics such as big data or artificial intelligence to clinical medicine data science for the very first time, video lectures by faculty in various fields introducing examples of data science in a variety of academic disciplines are incorporated into the curriculum.
Currently, a total of 13 video lectures are available, covering examples from general topics such as big data and artificial intelligence, to clinical medicine and sports.
This content helps motivate students from various background to learn and improves educational effectiveness.
The video curriculum is available for anyone to view on the Internet as OCW, with plans for expanded materials in the future.
For further details, see the section on e-Learning.
Abilities that can be gained through this educational program
Through lectures and practice on data utilization, students experience the entire data life cycle, including setting analysis goals, collecting data, preprocessing, analysis, and feedback of analysis results.
Doing so allows students to independently analyze various problems in society and develop the skills to make improvements for a better society.
Lectures on AI provide the knowledge required to survive in a society predicated on AI.
Implementation structure
Committee | Role |
Information Technical Committee Chair | Program operations manager |
Information Technical Committee | Program planning, enhancement, restructuring, improvement, and fixed schedule creation |
Information Promotion Office | Establishing and executing the curriculum |
Courses Offered and Study Details
Completion requirements
Acquisition of three credits in the two subjects of Information Literacy (lecture) (one credit) and Data Science (two credits).
Credit requirements
- Information Literacy (lecture): Graded from A+ to D on overall performance based on tests and reports.
Credits may be obtained with a grade of C and above. - Data Science: Graded from A+ to D on overall performance based on tests and reports.
Credits may be obtained with a grade of C and above.
Courses offered | Study details |
Information Literacy (lecture) | Students learn the basic concepts of information and the positioning of computers and the Internet in society in addition to the principles and configuration of computers, the principles of software, and the structure of the Internet. They also learn about information ethics, information security, and intellectual property necessary for safe and meaningful use of the Internet. |
Data Science | Students learn the basic concepts of data science and basic data analysis techniques using computers. They also practically acquire methods for understanding and utilizing data through data collection, data management, data visualization, and data analysis. The course touches upon advanced data science examples for an understanding of specific data uses in society. |
Class method
Information Literacy (lecture) and Data Science are compulsory subjects for all first-year students.
To ensure that all students can complete the course, Information Literacy (lecture) is offered in 27 Japanese classes and 1 English class (click here for a list of open subjects, Japanese only).
Data Science is offered in 50 Japanese classes and 1 English class (click here for a list of open subjects, Japanese only).
The Data Science model curriculum is offered in three levels so that the difficulty matches the student’s abilities.
Course materials are created to be compatible with all bachelor programs, not only science and engineering tracks, but for all subjects, and to meet the needs of society.
Furthermore, assessment of educational effectiveness for all students who have completed the course, student questionnaires, lecturer questionnaires, and course material feedback from lecturing faculty are used to design course materials to accommodate students who are unfamiliar with data science, those that wish to engage in more advanced studies, and students in a variety of disciplines.
Compatibility with Model Curriculum
Class content and elements | Class overview | ||||
(1) Things that are closely related to changes in society that are currently under way (Fourth Industrial Revolution, Society 5.0, data-driven society, etc.), and are closely linked to one’s life – Applies to model curriculum introduction 1-1, introduction 1-6 |
Students learn about data science that is closely linked to their daily lives. The expertise of faculty at the University of Tsukuba is used to learn about a broad range of fields where data science is utilized, such as information, medicine, sports, society, art, weather, and disaster prevention in order to increase motivation for data science endeavors. Using the three video lectures Big Data and IoT/CPS (Cyber-Physical System), Artificial Intelligence and Machine Learning, and Instant Tsunami Height Prediction in a Data-driven Society along with Modern Soccer Ball Aerodynamic Properties and Using Data to Excel at Soccer, students can learn about data science through familiar topics. As touched upon during the lectures, these video lectures can be viewed by students at any time. The program also touches upon Society 5.0, teaching students how data is used in our own society. | ||||
Class subject | Lecture theme | ||||
Data Science | Big Data, IoT, AI, and Robots (1, 5, 10) | ||||
Data Science | Increasing Amounts of Data (1) | ||||
Data Science | The Fourth Industrial Revolution, Society 5.0, and Data-driven Society (1) | ||||
Data Science | AI Services Combining Multiple Technologies (10) | ||||
Data Science | The Relationship Between Human Intellectual Activities and AI (10) | ||||
Data Science | How to View the World Through Data, How to View the World Through Human Intellectual Activities (1, 10) | ||||
Data Science | New Business Models Using AI (10) | ||||
Data Science | Case Studies on the Latest AI Technologies (1, 5, 10) | ||||
Information Literacy (lecture) |
Improving Computing Processing Performance (7) | ||||
(2) Data utilized in society and data utilization domains covers an extremely wide range, and can serve as a useful tool in everyday life and for solving social issues – Applies to model curriculum introduction 1-2, introduction 1-3 |
Class overview | ||||
Students acquire the knowledge required for group and sample data analysis, and learn the differences between complete surveys and sample surveys. They also learn the importance of random sampling in sample surveys. Students are requested to offer their own data, which is appropriately anonymized by lecturers to be used in data analysis exercises for students. In the second half of the Data Science class, data analysis is conducted as an exercise with the requisite preparations. Through such exercises, students learn the differences between primary and secondary data, how to create data, and data preparation methods. Students formulate a hypothesis on their own and use the government statistics service e-Stat to search for data required in analysis, analyze the data, verify their hypothesis, and engage in knowledge discovery. The video lecture Life Sciences and Data Science can also be taken to acquire advanced information. | |||||
Class subject | Lecture theme | ||||
Data Science | Survey Data, Experimental Data, Human Behavior Log Data, Machine Operation Log Data, etc. (1, 2, 3, 4, 5) | ||||
Data Science | Primary Data, Secondary Data, Metadata (2, 3, 4) | ||||
Information Literacy (lecture) |
Structured Data, Unstructured Data (6) | ||||
Data Science | Data Creation (2, 3, 4) | ||||
Data Science | Open Data (3) | ||||
Data Science | Expanding Realms of Data and AI Utilization (2, 3, 4) | ||||
Data Science | Hypothesis Verification, Knowledge Discovery, Cause Investigation, Planning, Judgment Support, etc. (1, 6, 7, 8, 9, 10) | ||||
(3) Various examples of on-site data utilization are shown and value is created by combining different application areas (logistics, manufacturing, finance, services, infrastructure, public domain, healthcare, etc.) with knowledge – Applies to model curriculum introduction 1-4, introduction 1-5 |
Class overview | ||||
Students learn about various fields in data science, such as information science, medicine, etc., from University of Tsukuba faculty and through video lectures. Specifically, in the initial stage of lectures, students may learn selectively from video lectures such as Human Interaction, Digital Humanities—A New World of Knowledge Derived From the Conjunction of Humanities and Information Science, Clinical and Social Medicine and Data Science—From the Viewpoint of Health Service Research, Cesium 137 Global Database, Environmental Radiation Data Searches and Public Websites, and Data Science and the Social Sciences. Students learn about the data analysis cycle, and how it consists of setting goals, analysis plans, data design, and data collection. Students not only learn theory, but also actual engagement in the cycle forming a hypothesis, collecting, analyzing, visualizing, and considering data during exercises. Students also analyze the data they collect themselves in preparation for application in the real world. | |||||
Class subject | Lecture theme | ||||
Data Science | Data Analysis: Prediction, Pattern Discovery, Optimization, and Simulation (1, 6, 7, 8, 9, 10) | ||||
Data Science | Data Visualization: Combination Graphs, Dual-axis Graphs, Multidimensional Visualization, and Visualization of Relationships (6, 7, 8, 9) | ||||
Data Science | Artificial Narrow Intelligence, Artificial General Intelligence, What AI Can and Cannot Do Now, AI and Big Data (10) | ||||
Data Science | Recognition Technology, Automated Technology (10) | ||||
Data Science | Data Science Cycles (9) | ||||
Data Science | Case Studies of Data and AI Usage in Services, Infrastructure, the Public Domain, and Healthcare (1, 5, 10) | ||||
(4) Students consider various key points in utilization (ELSI, personal information, data ethics, AI social principles, etc.), and learn about key points in protecting data, such as information security and information leaks – Applies to model curriculum guideline 3-1, guideline 3-2 |
Class overview | ||||
Students learn about information security technologies essential to our daily lives. This includes the importance of setting appropriate passwords and encryption technologies such as SSL/TLS. They deepen their understanding of cyberattacks that are now growing social issues, such as spam messages, malware, phishing, and DDoS attacks, the dangers inherent in using the Internet, and particularly the dangers in information leaks, as well as methods for safe use of the Internet. The class also covers personal information protection laws, the EU’s General Data Protection Regulation (GDPR), research ethics, ethical, legal, and social issues such as research and data ethics, and the importance of data handling in data utilization. Students also learn about alias and anonymizing technologies which are important for safe usage of collected data. The video lecture Ethical, Legal, and Social Issues in Artificial Intelligence covers the issues that come with the utilization of AI. | |||||
Class subject | Lecture theme | ||||
Data Science | ELSI(Ethical, Legal and Social Issues) (1) | ||||
Data Science | Personal Information Protection, EU General Data Protection Regulation (GDPR), Forgotten Rights, Opt-out (2) | ||||
Data Science | Data Ethics: Data Fabrication, Falsification, and Privacy Protection (2) | ||||
Data Science | AI Social Principles (fairness, accountability, transparency, human-centered decisions) (1) | ||||
Data Science | Data Bias, Algorithm Bias (1, 2) | ||||
Data Science | AI Service Responsibility Theory (1) | ||||
Information Literacy (lecture) |
Plagiarism (3) | ||||
Information Literacy (lecture) |
Information Security: Confidentiality, Completeness, Availability (3) | ||||
Information Literacy (lecture) |
Anonymously Processed Information, Encryption, Passwords, Malicious Information Exploitation (3)) | ||||
Information Literacy (lecture) |
Case Studies on Information Security Leak Incidents (3) | ||||
(5) Exercises using actual data and issues (including academic data) as actual materials in society on subjects related to fundamental use of mathematics, data science, and AI in reading, explaining, and handling data – Applies to model curriculum fundamentals 2-1, fundamentals 2-2, fundamentals 2-3 |
Class overview | ||||
Students learn about data types, such as qualitative and quantitative variables, the general theory behind selecting visual attributes and graphs, as well as various data visualization methods such as histograms, scatter diagrams, and line graphs. Inadequate visualization examples are also covered for reference when engaging in data visualization on their own. After learning how to create and visualize frequency distribution tables for qualitative data, students study cross-tabulation tables and the odds ratio. After learning how to create histograms for quantitative data, students learn the differences between average, median, and mode values, and descriptive statistics values such as distribution and standard deviations. They also learn how to calculate and interpret the correlation coefficient between two sets of data, as well as visualization methods for time-series data such as the running average and indexation. The class covers the relationship between two sets of data and instruction on how to understand the difference between correlation and causal correlation. In particular, students learn about spurious correlation, inverse correlation, and coincidental matches from actual events of note in the real world. ビThe video lecture Introduction to Hypothesis Testing can be viewed for repetitive learning at any time. | |||||
Class subject | Lecture theme | ||||
Data Science | Data Types (quantitative and qualitative variables) (2) | ||||
Data Science | Data Distribution (histograms) and Representative Values (average, median, and mode values) (6, 7) | ||||
Data Science | Differences in Representative Value Characteristics (In many cases, the average value does not equal the mode value in the real world) (7) | ||||
Data Science | Data Variability (distribution, standard deviation, deviation) (1, 7) | ||||
Data Science | Handling Errors Included in Observational Data (1, 3) | ||||
Data Science | Data Including Censoring and Omissions, Data Required for Stratification (1, 3) | ||||
Data Science | Correlation and Cause (correlation coefficient, spurious correlation, confounding) (1, 8, 9) | ||||
Data Science | Parent Population and Sample Selection (national census, questionnaire surveys, complete surveys, simple random sampling) (1, 2) | ||||
Data Science | Cross-tabulation Tables, Contingency Tables, Correlation Coefficient Matrices, Scatter Plot Matrices (6, 8) | ||||
Data Science | Correct Understanding of Statistical Information (Do not be misled by exaggerations) (6) | ||||
Data Science | Data Expression (bar graphs, line graphs, scatter diagrams) (6, 7, 8, 9) | ||||
Data Science | Data Diagram Expressions (creating charts) (6) | ||||
Data Science | Data Comparison (comparison with the requisite conditions) (7) | ||||
Data Science | Inadequate Graph Expressions (unnecessary visual elements) (6) | ||||
Data Science | Case Studies of Superior Visualizations (Examples of visualization resulting in new realizations) (9) | ||||
Data Science | Data Tallying, Rearrangement, Ranking, and Analysis Tools (2-9) |
Details and overview of the classes that make up the program (equivalent to selecting the mathematics, data science, and AI (literacy level) model curriculum) are as follows:
Class content and elements | Class subject |
Fundamentals of statistics and mathematics | Data Science |
Algorithm fundamentals | Information Literacy (lecture) |
Data structure and programing fundamentals | Information Literacy (lecture) |
Time-series data analysis | Data Science |
Data handling | Data Science |
Data usage practice (supervised learning) | Data Science |